Identify model vulnerabilities with AI Validation
Automatically test AI models for security and safety risks.
Get a Demo
Model validation automates AI security and safety testing
AI Validation performs a comprehensive assessment of security and safety vulnerabilities so you can understand the risks and protect against them. Our process uses algorithmic AI red teaming, which sends thousands of inputs to a model and automatically analyzes the susceptibility of the outputs across hundreds of attack techniques and threat categories using proprietary AI. It then recommends the necessary guardrails required to deploy safely in production, enforced by our AI Protection.
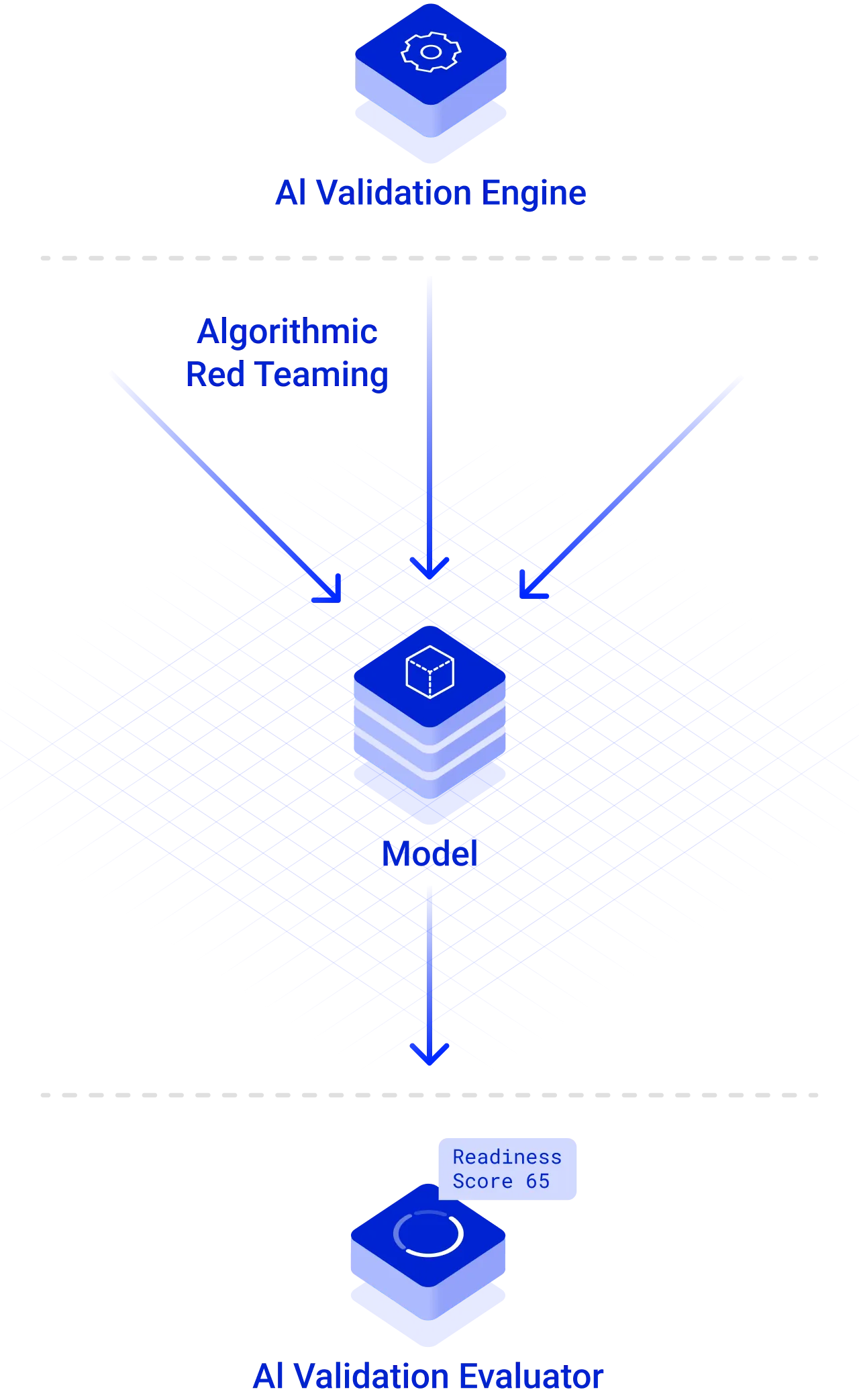
Integrates seamlessly into your workflows
AI Validation integrates into your CI/CD workflows, running unobtrusively in the background to enforce AI security standards across your organization. When a new model is entered into your model registry, an assessment can be automatically initiated via a simple API. Developers can also incorporate validation independently within their model development environment via our SDK.
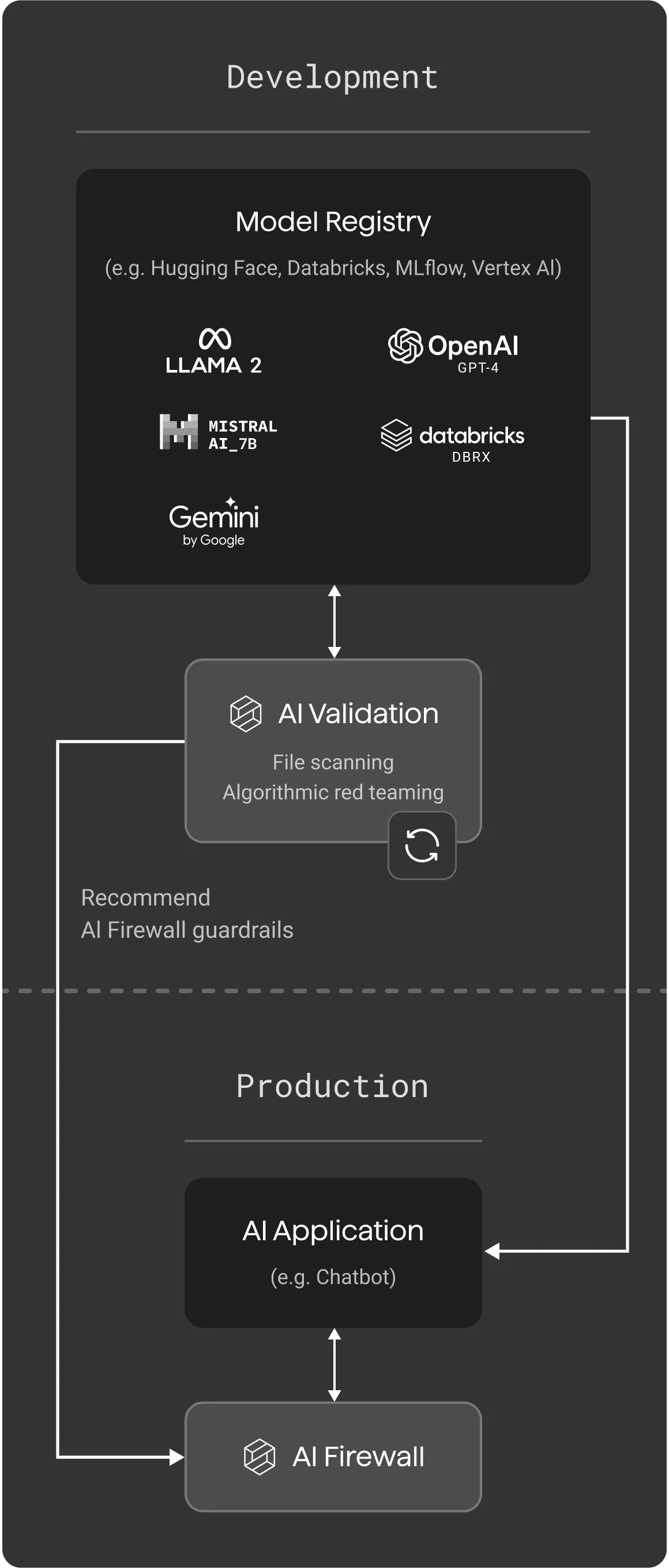
Automate AI security,
from development to production
Once an initial model assessment is completed, AI Validation carries out additional processes to ensure that your models are used securely and safely.
Guardrails recommendations
Automatically generate the guardrails that will protect the AI application using the specific vulnerabilities found in the model.
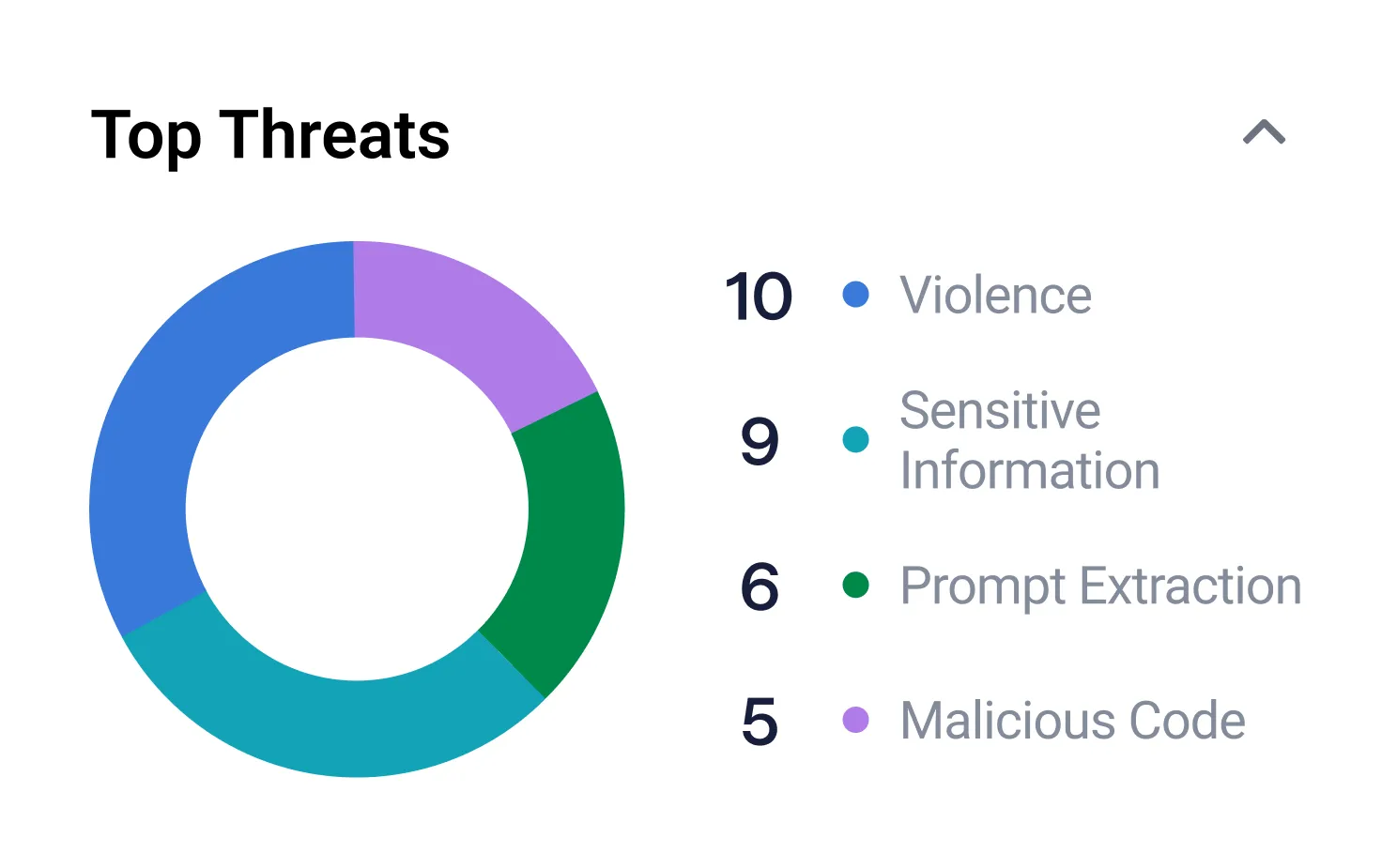
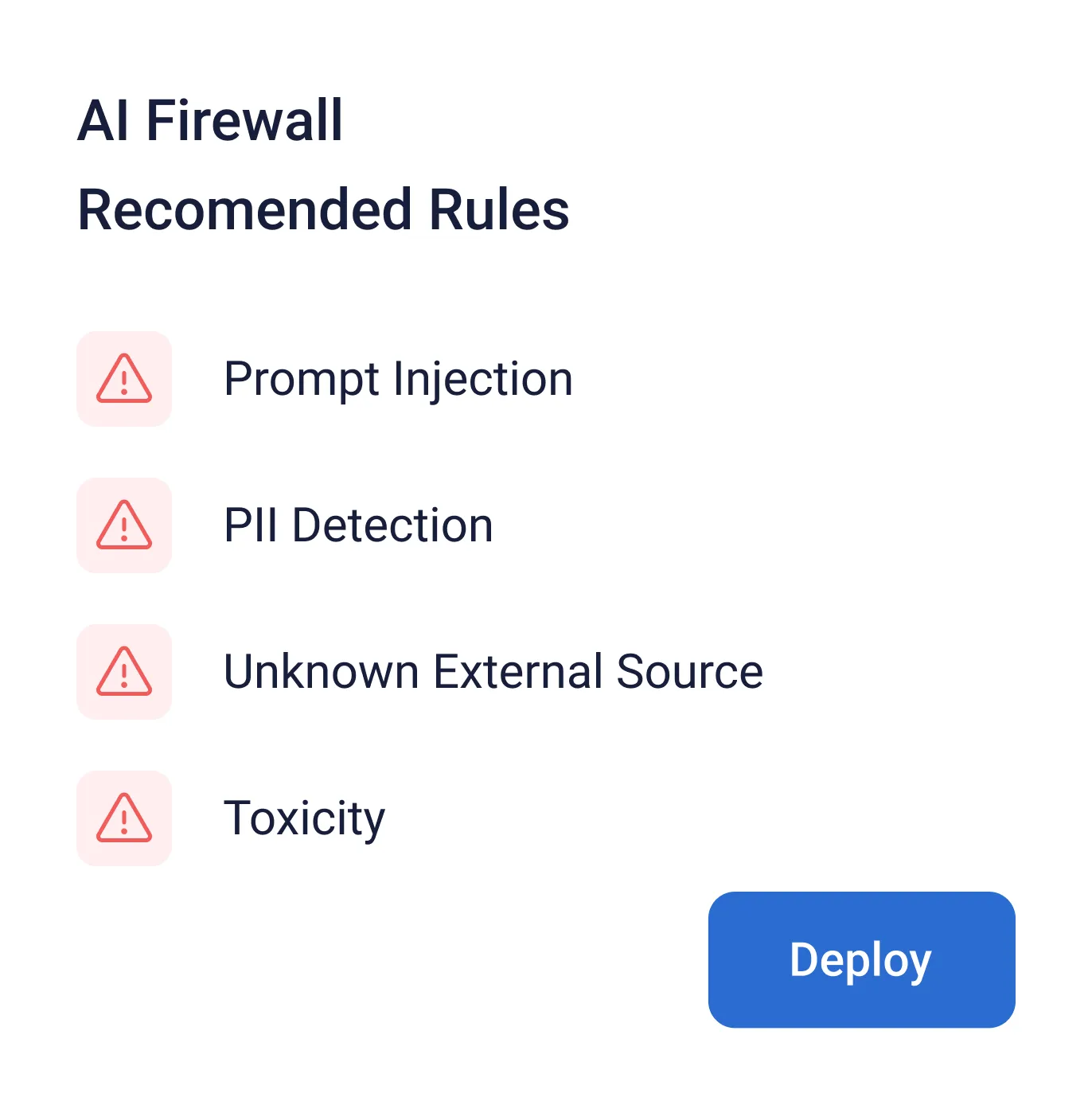
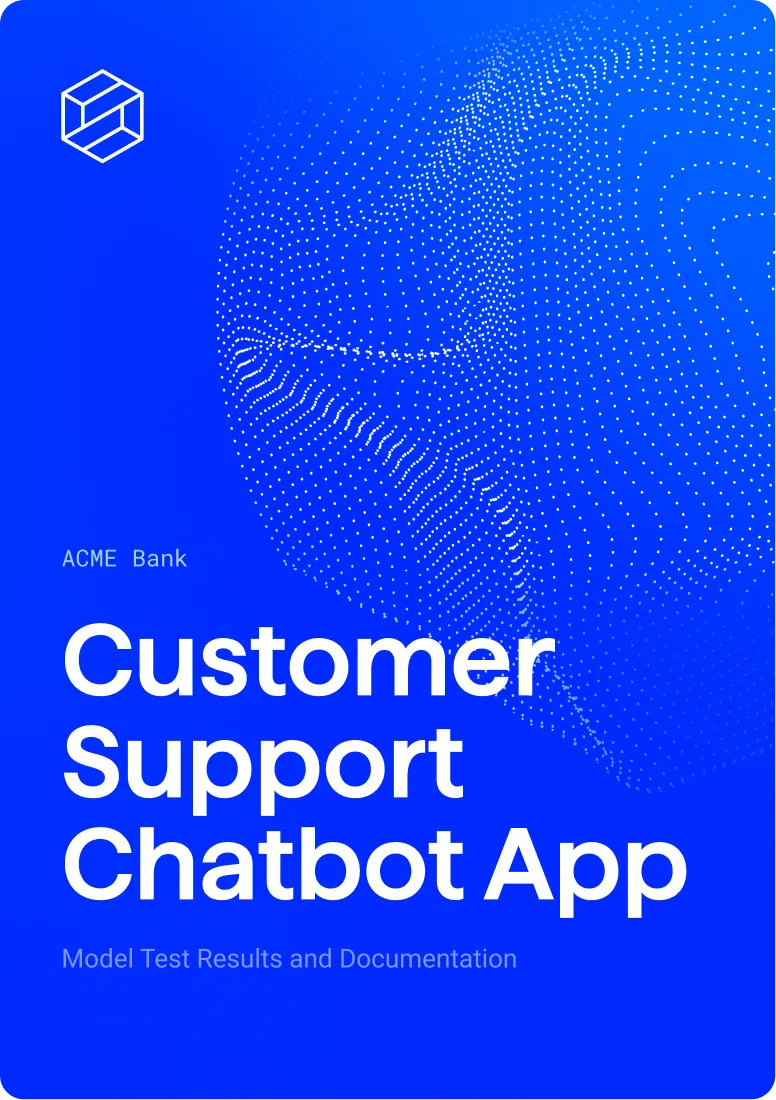


Reporting
Automatically generate model cards that translate the test results into an easy-to-read report that is mapped to industry and regulatory standards.
Continuous updates
Identify novel vulnerabilities with regular updates to our AI Validation test suite. This benefit extends to models in production, enabling the automatic discovery and “patch” of new vulnerabilities in existing models.

Achieve AI security excellence in your organization
Robust Intelligence makes it easy to comply with AI security standards, including the OWASP Top 10 for LLM Applications. This starts with automatically scanning open-source models, data, and files to identify supply chain vulnerabilities, such as malicious pickle files that can allow for arbitrary code execution. Our algorithmic testing covers the rest.
AI Validation Coverage | AI Protection Coverage | |
---|---|---|
LLM 01: Prompt injection attacks | ||
LLM 02: Insecure output handling | Not applicable | |
LLM 03: Data poisoning checks | Not applicable | |
LLM 04: Model denial of service | ||
LLM 05: Supply chain vulnerabilities | Not applicable | |
LLM 06: Sensitive information disclosure | ||
LLM 07: Insecure plug-in design | Not applicable | |
LLM 08: Excessive agency | Not applicable | |
LLM 09: Overeliance | ||
LLM 10: Model theft | Not applicable |
OWASP Top 10 for LLM Applications | ||||
---|---|---|---|---|
LLM 01: Prompt injection attacks | AI Validation Coverage | AI Protection Coverage | ||
LLM 02: Insecure output handling | AI Validation Coverage | AI Protection Coverage | Not applicable | |
LLM 03: Data poisoning checks | AI Validation Coverage | AI Protection Coverage | ||
LLM 04: Model denial of service | AI Validation Coverage | AI Protection Coverage | ||
LLM 05: Supply chain vulnerabilities | AI Validation Coverage | AI Protection Coverage | ||
LLM 06: Sensitive information disclosure | AI Validation Coverage | AI Protection Coverage | ||
LLM 07: Insecure plug-in design | AI Validation Coverage | AI Protection Coverage | Not applicable | Not applicable |
LLM 08: Excessive agency | AI Validation Coverage | AI Protection Coverage | Not applicable | |
LLM 09: Overeliance | AI Validation Coverage | AI Protection Coverage | ||
LLM 10: Model theft | AI Validation Coverage | AI Protection Coverage | Not applicable |
Adhere to AI standards' pre-production testing requirements
“...conduct AI red-teaming tests to enable deployment of safe, secure, and trustworthy systems.”
“...incorporate secure development practices for generative AI [and] guidance [for] successful red-teaming.”
“...mandatory requirements for trustworthy AI and follow conformity assessment procedures...”
Trust that your models are secure and safe
AI Validation uses over 150 security and safety categories when performing algorithmic testing of models, which can find vulnerabilities to malicious actions, such as prompt injection and data poisoning, or unintentional outcomes. There are four primary failure categories:
Abuse Failures
Toxicity, bias, hate speech, violence, sexual content, malicious use, malicious code generation, disinformation
Privacy Failures
PII leakage, data loss, model information leakage, privacy infringement
Integrity Failures
Factual inconsistency, hallucination, off-topic, off-policy
Availability Failures
Denial of service, increased computational cost
Learn more about individual AI risks, including how they map to standards from MITRE ATLAS and OWASP, in our AI security taxonomy.
Test the models that power your application
AI Validation validates your models and recommends the guardrails to cover any vulnerabilities, thereby removing AI security blockers to deploy applications in minutes.
Foundation Models
Foundation models are at the core of most AI applications today, either modified with fine-tuning or purpose-built. Learn what challenges need to be addressed to keep models safe and secure.
RAG Applications
Retrieval-augmented generation is quickly becoming a standard to add rich context to LLM applications. Learn about the specific security and safety implications of RAG.