The rapid evolution and enterprise adoption of AI has motivated bad actors to target these systems with greater frequency and sophistication. Many security leaders recognize the importance and urgency of AI security, but don’t yet have processes in place to effectively manage and mitigate emerging AI risks with comprehensive coverage of the entire adversarial AI threat landscape.
In their 2023 CISO Village Survey, Team8 found that 48% of CISOs cited AI security as their most acute problem—the highest of any category. While AI itself is relatively nascent, the impact of a successful attack can be severe.
In order to successfully mitigate these attacks, it’s imperative that the AI and cybersecurity communities are well informed about today’s AI security challenges. To that end, we’ve co-authored the latest update to NIST’s taxonomy and terminology of adversarial machine learning.
Let’s take a glance at what’s new in this latest update to the publication, walk through the taxonomies of attacks and mitigations at a high level, and then briefly reflect on the purpose of taxonomies themselves—what are they for, and why are they so useful?
What’s new?
The previous iteration of the NIST Adversarial Machine Learning Taxonomy focused solely on predictive AI, models designed to make accurate predictions based on historical data patterns. Individual adversarial techniques were grouped into three primary attacker objectives: availability breakdown, integrity violations, and privacy compromise.
Availability breakdown attacks degrade the performance and availability of a model for its users.
Integrity violations attempt to undermine model integrity and generate incorrect outputs.
Privacy compromises infer or directly access sensitive data such as information about the underlying model and training data.
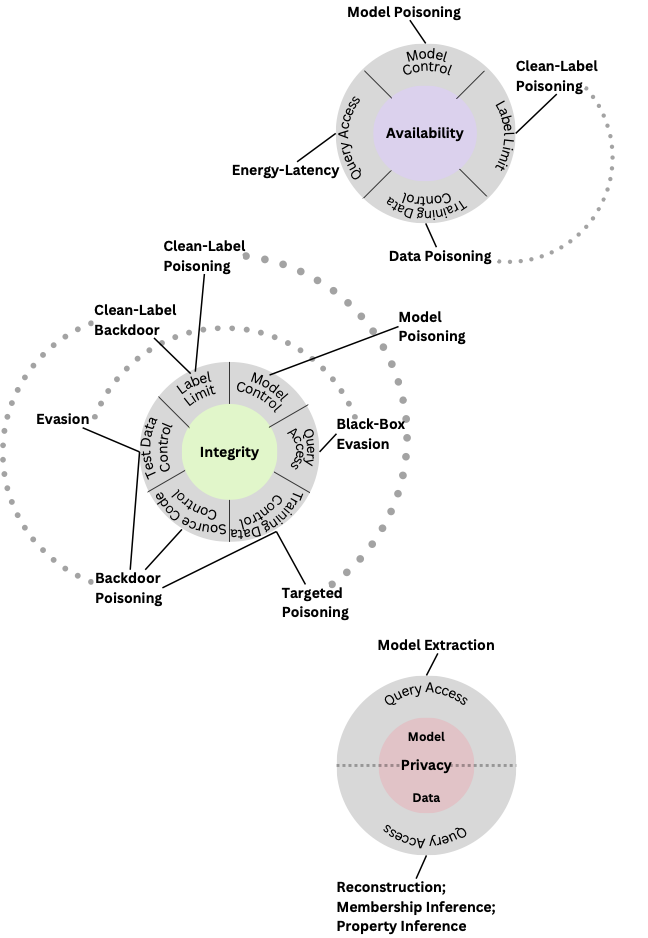
The most notable change in this revision is the addition of an entirely new taxonomy for generative AI models.
The new generative AI taxonomy inherits the same three attacker objectives as predictive AI—availability, integrity, and privacy—but with new individual techniques. There’s also a new fourth attacker objective unique to generative AI: abuse violations.
Abuse violations repurpose the capabilities of generative AI to further an adversary’s malicious objectives by creating harmful media or content that supports cyber attack initiatives.
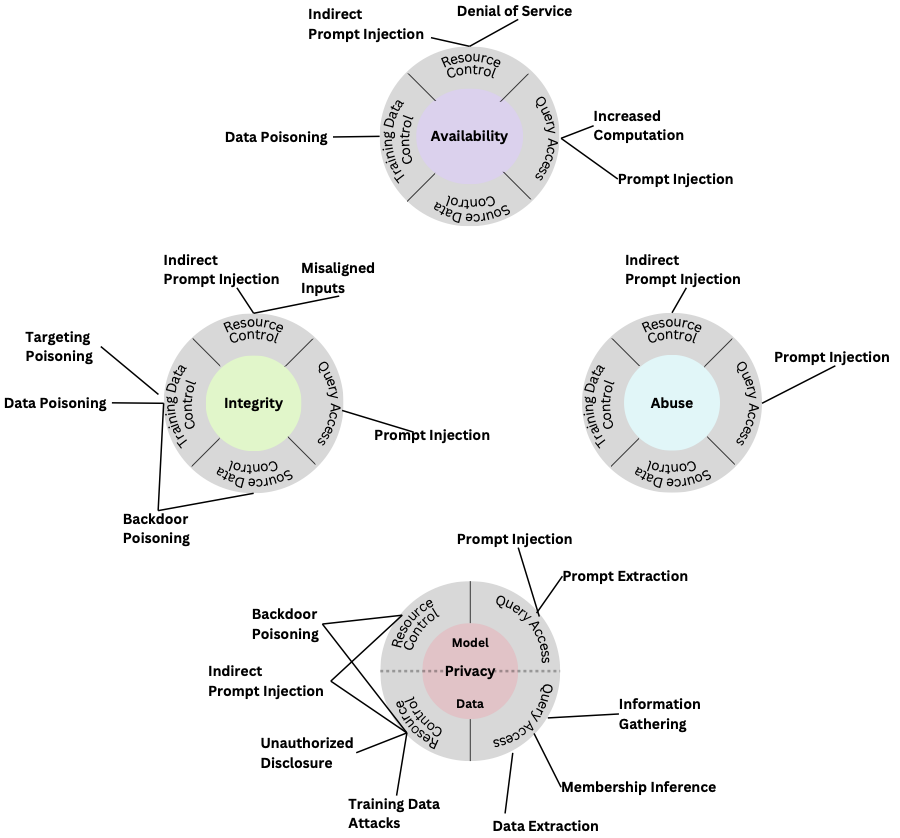
Classifying attacks on Generative AI models
As mentioned, the generative and predictive adversarial taxonomies share three common attacker objectives. The fourth objective, abuse, is unique to generative AI.
To achieve one or several of these goals, adversaries can leverage a number of techniques. The introduction of the generative AI section brings an entirely new arsenal that includes supply chain attacks, direct prompt injection, data extraction, and indirect prompt injection.
Supply chain attacks are rooted in the complexity and inherited risk of the AI supply chain. Every component—open-source models and third-party data, for example—can introduce security issues into the entire system.
These can be mitigated with supply chain assurance practices such as vulnerability scanning and validation of datasets.
Direct prompt injection alters the behavior of a model through direct input from an adversary. This can be done to create intentionally malicious content or for sensitive data extraction.
Mitigation measures include training for alignment and deploying a real-time prompt injection detection solution for added security.
Indirect prompt injection differs in that adversarial inputs are delivered via a third-party channel. This technique can help further several objectives: manipulation of information, data extraction, unauthorized disclosure, fraud, malware distribution, and more.
Proposed mitigations help minimize risk through reinforcement learning from human feedback, input filtering, and the use of an LLM moderator or interpretability-based solution.
What are taxonomies for, anyways?
Hyrum Anderson, our CTO, put it best when he said that “taxonomies are most obviously important to organize our understanding of attack methods, capabilities, and objectives. They also have a long tail effect in improving communication and collaboration in a field that's moving very quickly.”
It’s why our team at Robust Intelligence strives to collaborate with leading organizations like NIST, and to aid in the creation and continuous improvement of shared standards.
These resources give us better mental models for classifying and discussing new techniques and capabilities. Awareness and education of these vulnerabilities facilitate the development of more resilient AI systems and more informed regulatory policies.
You can review the entire NIST Adversarial Machine Learning Taxonomy and learn more with a complete glossary of key terminology in the full paper, available here.