“NTT DATA is aiming to deploy high-performance and safe AI models by standardizing the AI development process. Using the RIME platform, we were assisted in the quality control process (which previously was carried out manually) and proceeded with development more efficiently, robustly, and uniformly. The technical skills of the Robust Intelligence engineers are extraordinary. They have built a trusted relationship with the NTT DATA team due to the efficiency and sincere manner that they respond to our requests.” –– Eiji Yoshida, Head of IOWN Innovation Office
NTT DATA is a top 10 global IT services provider operating in more than 50 countries and headquartered in Tokyo, Japan. Since its founding in 1967, NTT DATA has played a crucial role in establishing and advancing global IT infrastructure. Companies are increasingly using AI to optimize business processes, gather insights and establish proof-of-concepts (POCs) for new businesses. To do this safely, companies must consider the risks associated with AI models and manage data inputs and model vulnerabilities to enhance decision-making capabilities. NTT DATA uses RIME, our solution to eliminate operational risks in AI, to strengthen AI governance and achieve a more accurate model. A version of this case study is also available in Japanese on their website (press release, blog post).
Why AI Robustness is a high priority for NTT DATA
NTT DATA is integrating AI into critical automated decision processes with increasing frequency. However, there is a risk associated with scaled AI, as it depends on insufficient training data and models that are extrapolated beyond their designed use. Without a method to predict with certainty how AI will operate with data outside of training datasets, there are significant security risks to the entire system due to unexpected model behaviors. Further, there have been reports of malicious users discovering model vulnerabilities in AI systems, which are intentionally exploited to cause malfunctions. For these reasons, NTT DATA feels a strong necessity to pinpoint critical weaknesses that lead to vulnerabilities (Fig.1) during the development stage of AI and take preventative measures against negative high-impact outcomes. Figure 1 shows typical examples of AI dataset and model risks NTT DATA sees.
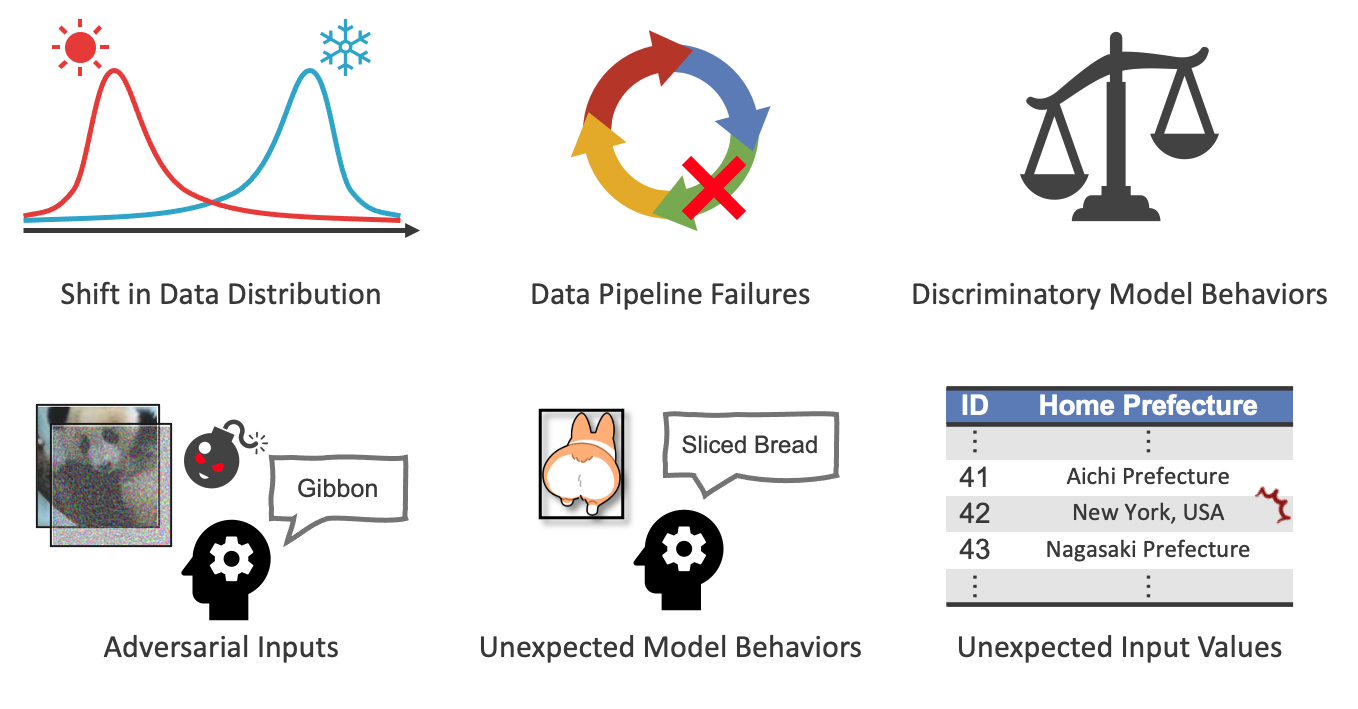
NTT DATA have advanced their data analytics capabilities by using AI and use this optimization of data insights to help build IT solutions for their customers. The application of NTT DATA’s AI-based advanced analytics spans across many applications – healthcare diagnostic imaging, business intelligence, manufacturing automation, smart cities, and financial services. In integrating the use of AI to advance and enhance data analytics solutions, NTT DATA is prioritizing the initiative to strengthen AI governance.
Why NTT DATA chose Robust Intelligence
Unfortunately, identifying every weakness in AI is not an easy task. This is because there is an infinite amount of unknown data, and every behavior related to this data cannot be verified. Currently, business experts, data scientists, and other highly skilled professionals are carrying out multi-faceted verifications in AI models one by one to identify as many weaknesses as possible. However, this approach is strongly dependent on the person's skills and requires high costs to implement. RIME offers a solution to these types of problems inherent with AI models.
NTT DATA’s initiative, “Strengthen AI Governance," has been utilizing RI’s solution to perform technical verifications for AI security since 2020. These technical verifications used RIME to detect and address vulnerabilities in our deep learning-based Image Similarity Search Model for trademark images. This use case is used as a way to automate manual searches for copyright trademarks. The model’s fragility causes problematic outputs, hence requiring a third-party check system such as what RIME offers. RIME offers model stress testing and uses RI’s AI Firewall™ so that the image search returns the correct output despite some model fragility.
How RIME secures NTT DATA Models
The Image Similarity Search Model searches for similar images in a 500,000+ previously registered trademark images database. This model aims to improve work efficiency by allowing users to upload any proposed logo image and confirm whether there is a similar trademark image in the database.

By utilizing this model, NTT DATA reduces unnecessary trademark applications since companies can confirm whether a similar image exists before applying for a trademark. On the other hand, they must be aware of the possibility of malicious attacks and unexpected input data when utilizing this model to verify that they protect the rights of previous applicants. For example, they can expect that inexperienced users input mismatched data into system requirements or malicious attackers intentionally cause malfunctions that may degrade the reliability of search results. To examine these vulnerabilities, they used RIME to test the model’s behaviors when presented with various images.

Discover model weaknesses with AI Stress Test
This verification process utilized the RIME AI STRESS TEST function to perform various tests and measure the robustness of this model. Based on the prediction results of these inputs, RIME applied additional transformations to induce misclassification further.

The results of this verification process showed that the Image Similarity Search Model was robust to color changes. However, the search performance degraded when images were rotated or when particular noise was added.

Prevent model failures with AI Firewall
After discovering the model weaknesses, NTT DATA improved their level of AI robustness by utilizing RIME AI FIREWALL to sanitize corrupted images. AI Firewall protects the AI model in production from data that can cause critical errors and is generated from failures identified by AI Stress Testing. Specifically, AI Firewall for images can take input images, detect the corruption that can result in model failures, and auto-correct them so that the resulting output remains valid.

For example, based on the AI Stress Testing result that NTT DATA model is vulnerable to particular noise, RIME auto-generated an AI Firewall that can effectively remove the noise from a candidate image. This portion of the AI Firewall significantly outperforms off-the-shelf denoisers, such as the image denoiser from OpenCV. Unlike off-the-shelf denoisers, AI Firewall is customized: we show the denoiser examples from the NTT DATA Copyright dataset, from which it learns the distribution of clean images, which enhances its ability to recognize noise when present. The following is a qualitative comparison of our image denoiser and the OpenCV implementation of the non-local means denoising algorithm.

Based on AI Firewall, NTT DATA was able to improve their similarity search model performance by almost 70%.
Prevent model failures with AI Firewall
RIME detected and prevented many vulnerabilities in NTT DATA AI models, resulting in model performance improvements. After our success with the image models, NTT DATA has already started applying RIME to their tabular models and plans to apply it to natural language models as well. RI will continue to protect a broad range of AI applications at NTT DATA.