Today, the National Institute of Standards and Technology (NIST) published the highly anticipated AI Risk Management Framework (AI RMF) 1.0 and corresponding NIST AI RMF Playbook (among a few other accompanying resources). This is the culmination of 15 months of work, including three public workshops and two rounds of drafts. The AI RMF is a voluntary guideline intended to help companies effectively navigate the business risk that comes with AI adoption.
As a preeminent physical science laboratory attached to the U.S. Department of Commerce, NIST standards are designed to enhance economic security and improve our quality of life. The issue of AI risk management is a pressing concern for companies. As outlined by NIST,
In collaboration with the private and public sectors, NIST has developed a framework to better manage risks to individuals, organizations, and society associated with artificial intelligence (AI). The NIST AI Risk Management Framework (AI RMF) is intended for voluntary use and to improve the ability to incorporate trustworthiness considerations into the design, development, use, and evaluation of AI products, services, and systems.
The U.S. Congress recognized this need for guidance in AI risk management and directly assigned the task to develop this voluntary framework to NIST. NIST has quickly acted on this congressional mandate and produced a comprehensive framework that will help shape the future of AI adoption.
What is AI risk management and why is it important?
AI and machine learning are now considered key factors for companies in driving competitive advantage. Intelligent automation helps companies improve their efficiency by reducing costs and improving the quality of products offered to customers – across all industries. AI is being applied to a wide variety of applications such as evaluating levels of risk, calculating credit scores, fraud detection, loan default prediction, insurance underwriting, personalizing banking experiences, and more.
This innovation also opens companies to AI risk. When models fail making business-critical decisions, the consequences can be harmful to the business and consumer. Therefore, it’s imperative that companies adopt strategies to manage and mitigate AI risk. According to NIST’s AI RMF 1.0 (page. 4),
AI risk management offers a path to minimize potential negative impacts of AI systems, such as threats to civil liberties and rights, while also providing opportunities to maximize positive impacts. Addressing, documenting, and managing AI risks and potential negative impacts effectively can lead to more trustworthy AI systems.
As the application of AI continues to grow, so do the challenges and risks that arise in the use of AI-driven techniques. AI models are more difficult to manage and maintain that traditional statistical models, which can lead to erroneous predictions unless they’re addressed by instilling integrity in your machine learning systems. Below are some of the primary challenges to managing AI risk:
1. AI models are black boxes
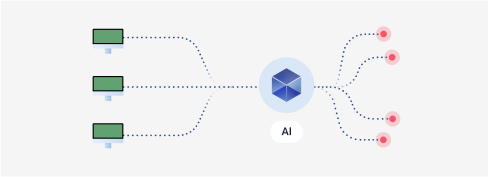
It can be far more difficult to measure the robustness of AI models than it is for traditional statistical models. Traditional models have various parameters used in training, but each one of these has explainable assumptions and leads to predictable results. However, AI models don’t follow a linear decision making process and have limited interpretability. For example, financial institutions might not be able to reveal the mechanism behind their model creation or the data used to train such models in order to protect intellectual property or consumer privacy.
2. AI models are heavily dependent on data

AI models learn and improve from the data used in training. However, data provided to AI models is not always reliable. Unreliable data may include poorly labeled data, incomplete or missing data, duplicated data, data instances with outlier values from what the model was trained on, and differing distributions of data that the model is trained on as opposed to what it will see in production deployment. The tricky thing with AI models is that even when the models are taking in poor quality data inputs, they're not necessarily going to fail outright — they will simply produce erroneous outputs, creating a silent failure. This, combined with AI models being a black box, makes it harder to detect data abnormalities in AI-based applications.
3. Bias and discrimination
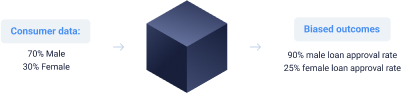
There is a strong possibility that bias and discrimination is introduced, and in fact intensified, in AI models. An AI-based loan approval model can introduce bias against a particular demographic based on race would violate several regulations and will be in conflict with the company’s financial inclusion goals. Discrimination can be a result of poor quality of data. Models can also unintentionally introduce bias through inference or proxies. For example, an algorithm can infer race and gender by evaluating location data and purchase activity.
4. Security

AI models are also susceptible to cyber attacks. Many models can be fooled by malicious actors into making incorrect predictions with virtually unnoticeable changes to the input, known as adversarial attacks. Data poisoning attacks by attackers can corrupt a model by training it with a flood of bad data to deliberately sabotage the model. Given that AI models are prone to such security attacks, it is critical to harden your model against such vulnerabilities and identify the most robust candidate models.
Taken together, the reason there is a push for enhanced AI risk management is that AI demands new and challenging ways to manage associated risk that haven’t been needed before with traditional statistical modeling. AI models are far more complex, prone to overfitting because of their dependence on historical data, potential to amplify existing human biases, and susceptibility to security risk (to name some of the key reasons). NIST has recognized a need for a creating a unified and comprehensive framework for how companies can effectively manage these risks across the whole AI lifecycle.
Overview of NIST AI Risk Management Framework
NIST’s AI RMF is a comprehensive guideline comprised of four functions to manage AI risk: Govern, Map, Measure, and Manage (as illustrated below, NIST AI RMF 1.0 page. 20)
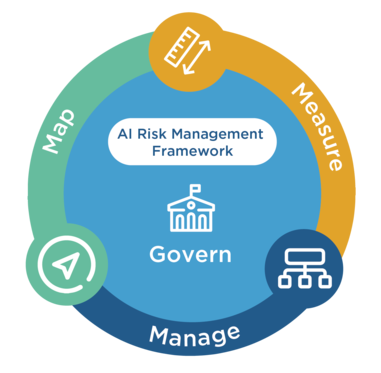
NIST has created this framework to incorporate each stage of the AI lifecycle, ensuring that risk management is captured from start to finish of the process of designing to deploying an AI system.
- The GOVERN function is at the core of the framework and it encourages the adoption of a culture of risk management within an organization. (page. 21)
- The MAP function “establishes the context to frame risks related to an AI system.” (page. 24)
- The MEASURE function “employs quantitative, qualitative, or mixed-method tools, techniques, and methodologies to analyze, assess, benchmark, and monitor AI risk and related impacts.” (page. 28)
- The MANAGE function “entails allocating risk resources to mapped and measured risks on a regular basis and as defined by the GOVERN function.” (page. 31)
Altogether, these functions aim to bring trust to AI technologies and to promote and normalize risk mitigation in AI innovation.
What can companies do to align with the framework?
NIST makes it clear that the NIST AI RMF 1.0 is aiming to reach a broad and diverse audience (page. 9),
Identifying and managing AI risks and potential impacts – both positive and negative – requires a broad set of perspectives and actors across the AI lifecycle. Ideally, AI actors will represent a diversity of experience, expertise, and backgrounds and comprise demographically and disciplinarily diverse teams. The AI RMF is intended to be used by AI actors across the AI lifecycle and dimensions.
Although the framework is intending to provide guidance and be voluntary, it will serve as an important benchmark for establishing the responsible use of AI and how relevant stakeholders should engage with the adoption of AI.
Robust Intelligence believes that the best way to adhere to the AI RMF and effectively manage AI risk is to implement a continuous validation approach. By incorporating automated testing of models and data across your ML pipeline, you can proactively mitigate model failure resulting from performance, fairness, and security issues.
To learn more about how our platform aligns with the NIST framework, we invite you to request a demo. Additionally, you can register for our webinar with one of NIST’s Principal Investigators, Reva Schwartz, on February 9th to learn more!